What is Data lakes and AI? How AI helps ?
September 30, 2024
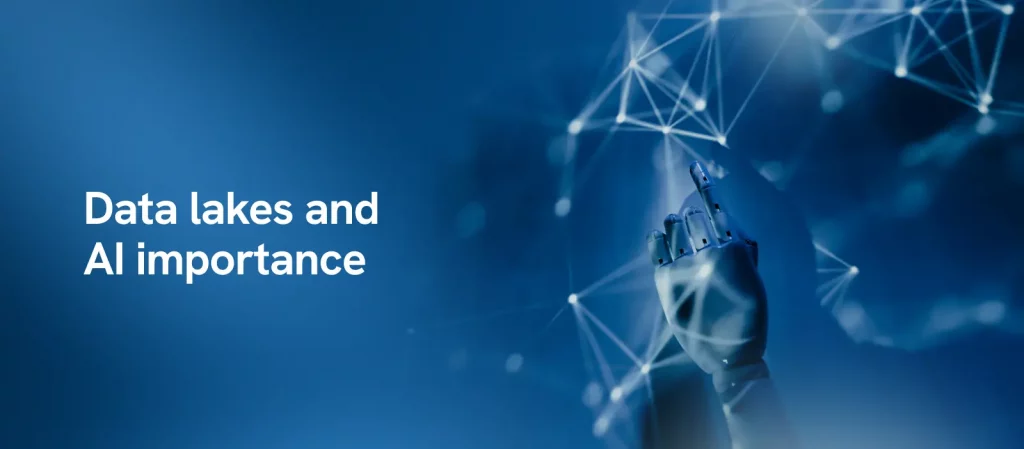
The world has become a data-driven world nowadays. Organizations increasingly rely on large-scale data lakes to store and process vast amounts of structured, semi-structured, and unstructured data.
A data lake is a centralized repository that allows you to store all your structured and unstructured data at any scale. Unlike traditional databases or data warehouses, a data lake stores raw data in its native format until it’s needed for processing.
At the same time, Artificial Intelligence (AI) and Machine Learning (ML) have become critical tools for analyzing this data, generating insights, and driving automation. When paired together, data lakes and AI open up new possibilities, transforming industries through enhanced decision-making, predictive analytics, and automation.
What is a Data Lake?
A data lake is a centralized repository that allows organizations to store all of their data, regardless of format or source, in one location. Unlike traditional databases or data warehouses, data lakes can handle raw, unprocessed data in its native format. This means that data from various sources—such as IoT devices, social media, business transactions, and legacy systems—can be ingested and stored without predefined schemas.
Data Lakes for Artificial Intelligence
Data lakes play a crucial role in providing large amounts of data to extract meaningful insights. The vast amount of unstructured and semi-structured data stored in a data lake is often too large for traditional analytical methods.
AI, especially through machine learning and deep learning, can process this data at scale, enabling organizations to perform advanced tasks such as:
Predictive Analytics: The AI models, that can analyze historical data to forecast future outcomes (e.g., sales trends, equipment failures).
Natural Language Processing (NLP): AI can analyze and understand large volumes of textual data, such as customer reviews or other documents.
Image and Video Recognition: AI models can process images and videos stored in the data lake to detect patterns, recognize objects, or perform sentiment analysis.
Let’s explore how data lakes, and AI, are being applied across different industries with real-world examples.
Healthcare: Predictive Diagnosis and Personalized Treatment
The healthcare provider created a data lake using Amazon S3 to store patient records, medical images, lab results, and genomic data. With this centralized repository, AI algorithms will be developed to predict patient health outcomes, enabling early diagnosis of diseases like diabetes and heart conditions.
How AI helps:
1. Predictive Analytics: AI analyzes historical medical data from millions of patients to predict potential risks of diseases and recommend preventive measures.
2. Personalized Treatment Plans: By analyzing a patient’s genomic data, medical history, and lifestyle patterns, AI can recommend personalized treatment plans that are more effective.
3. Medical Imaging: AI-driven computer vision models can analyze X-rays, CT scans, and MRIs to detect abnormalities (e.g., tumors) with high accuracy.
Impact: This combination of data lakes and AI has led to faster, more accurate diagnoses and more personalized care plans, improving patient outcomes while reducing costs for the healthcare provider.
Retail: Enhancing Customer Experience with AI-Powered Insights
Let’s consider a global retail chain that integrated its e-commerce, customer service, and point-of-sale systems into a data lake. This allowed them to aggregate customer interaction data across multiple channels—website visits, purchases, customer service chats, and social media interactions.
How AI helps:
1. Customer Behavior Analysis: AI models can analyze customer behavior patterns, predicting future purchases based on browsing history, past purchases, and product recommendations.
2. Sentiment Analysis: Using Natural Language Processing (NLP), AI can analyze customer reviews and social media posts, identifying potential issues or trends in customer sentiment.
3. Personalization: AI-driven recommendation engines suggest personalized product offerings to customers based on their preferences, browsing behavior, and purchase history.
Impact: The integration of data lakes and AI has significantly improved customer satisfaction through more personalized shopping experiences, leading to higher customer retention rates and increased sales.
Manufacturing: Predictive Maintenance and Quality Control
Let’s consider a large automobile manufacturer that developed a data lake to aggregate IoT data from thousands of sensors across its production facilities. The data included machine operational data, environmental conditions, and product quality metrics.
How AI helps:
1. Predictive Maintenance: By analyzing sensor data from manufacturing equipment, AI models can predict when machines are likely to fail or require maintenance. This helps reduce downtime and prevent costly repairs.
2. Quality Control: AI models process real-time data from production lines to detect defects or deviations in product quality. This allows for immediate corrective actions, reducing waste and improving product consistency.
3. Supply Chain Optimization: AI-driven insights from the data lake help optimize the supply chain by predicting demand, managing inventory, and reducing overproduction.
Impact: The use of AI for predictive maintenance and real-time quality control has improved efficiency, reduced downtime, and lowered operational costs, while ensuring high-quality standards are consistently met.
Financial Services: Fraud Detection and Risk Management
Let’s consider a major bank implementing a data lake to store transactional data, customer profiles, and unstructured data such as emails and social media interactions. AI algorithms analyze the data to detect fraudulent activities in real time.
How AI helps:
1. Fraud Detection: AI models can analyze transaction patterns in real time, identifying suspicious activities that could indicate fraud. This is particularly useful for detecting anomalies in credit card transactions or wire transfers.
2. Risk Management: AI-driven predictive models help assess credit risk by analyzing financial history, employment data, and even social media activity to determine an individual’s creditworthiness.
3. Chatbots and NLP: AI chatbots, powered by NLP, are integrated into customer service to handle basic banking inquiries, improving customer experience while reducing response time.
Impact: The bank saw a substantial reduction in fraud incidents, improved risk management, and enhanced customer service through AI-driven automation, all powered by its data lake infrastructure.
Also read: Wannacry Ransomware Attack: How to Protect Your Business?
The Future of Data Lakes and AI
The integration of data lakes and AI is transforming industries by enabling real-time decision-making, predictive analytics, and automation at scale. As organizations continue to adopt AI technologies, the role of data lakes will become even more critical, providing the foundation for AI-powered innovation.
Looking ahead, we can expect:
1. More Efficient AI Models: With access to richer, more diverse datasets, AI models will become even more accurate and efficient.
2. Edge Computing Integration: As IoT devices become more prevalent, data lakes will increasingly integrate with edge computing, allowing real-time processing at the data source.
3. Automated Data Governance: With tools like AWS Lake Formation and Azure Purview, AI can help automate data governance and compliance, ensuring that data in lakes remains secure and accessible.
In conclusion, the synergy between data lakes and AI is unlocking unprecedented possibilities for businesses across industries, helping them turn raw data into actionable insights and competitive advantage.
A few popular data lakes are available.
Several popular data lake platforms are available, each offering distinct features to help organizations store, manage, and analyze large volumes of structured and unstructured data. Here are some of the most widely used data lakes:
Amazon Web Services (AWS) – Amazon S3 (Simple Storage Service)
Overview: Amazon S3 is one of the most popular data lake solutions, especially for organizations already using AWS. It’s highly scalable, cost-effective, and integrates with other AWS services for analytics, machine learning, and data management.
Key Features:
1. Supports structured, semi-structured, and unstructured data.
2. Integrated with services like AWS Glue (for ETL), Amazon Athena (for querying), and AWS Lake Formation (for governance).
3. Scalable storage and compute separation.
Microsoft Azure – Azure Data Lake Storage (ADLS)
Overview: Azure Data Lake Storage is a scalable data lake built on Azure’s cloud platform. It is designed for high-performance analytics and integrates well with other Azure services like Azure Synapse Analytics.
Key Features:
1. Hierarchical namespace for better data organization and performance.
2. Integration with Azure Data Factory for data ingestion and ETL.
3. Built-in security with role-based access control and encryption.
Google Cloud – Google Cloud Storage (GCS)
Overview: Google Cloud Storage is a highly scalable and secure data lake platform that works seamlessly with Google’s suite of analytics and machine learning tools.
Key Features:
1. Integrates with BigQuery for querying large datasets and Google Cloud Dataflow for stream and batch data processing.
2. Strong integration with Google’s AI and machine learning products (e.g., TensorFlow).
3. Unified data model that allows easy handling of structured and unstructured data.
Cloudera Data Platform (CDP)
Overview: Cloudera Data Platform is an enterprise data lake solution offering multi-cloud, on-premise, and hybrid deployments. It’s built on Hadoop but supports modern data lake architectures.
Key Features:
1. Unified platform for data engineering, machine learning, and analytics.
2. Multi-cloud and hybrid architecture support.
3. Advanced data governance, security, and compliance capabilities.
Snowflake
Overview: Snowflake is a cloud data platform that provides a fully managed, scalable data warehouse that can act as a data lake for structured and semi-structured data.
Key Features:
1. Built for cloud environments (supports AWS, Azure, and Google Cloud).
2. Supports storage and querying of semi-structured data (JSON, Avro, Parquet).
3. Separation of computing and storage for cost efficiency.
Conclusion
Data lakes and AI, revamping how organizations manage, analyze, and utilize their data. These data lakes provide a scalable, cost-effective storage solution for structured, semi-structured, and unstructured data. When combined with AI and ML, this data can be leveraged to gain valuable insights, drive automation, and better personalization. Combining data lakes and AI enables businesses to make more informed decisions, personalize customer experiences, and improve their security. As more data is analyzed, the system’s accuracy and efficiency will improve, propelling businesses toward greater success.
Also, Read our blog about: How Integrating AI and machine learning into Salesforce Apps
Keep reading about
LEAVE A COMMENT
We really appreciate your interest in our ideas. Feel free to share anything that comes to your mind.