What we did?
- Advanced state-of-art AI models
- High-performance training of models
- Real-Time prediction and Decision Making
- User-friendly UI to manage the application
Now the client is able to successfully identify good service from bad service within seconds which would otherwise have taken a lot of human effort. Also based on the time series forecast of call data the client is able to predict future call volume and we are in the process of automating the downstream processes for better resource allocation.
Project Overview
The world is never interconnected before since the inception of Telecommunication Devices. With the entry of 5G, it is going to get even profound and a Gartner’s study states that the world’s 90% of the data has been generated in the last two years hence the IoT related Data and Analytics are in demand considering petabytes of data that is going to get generated in the future. Companies know this trend very well and started investing in this vertical more serious than ever. One such telecom based company approached Terralogic for AI solutions in their LTE business.
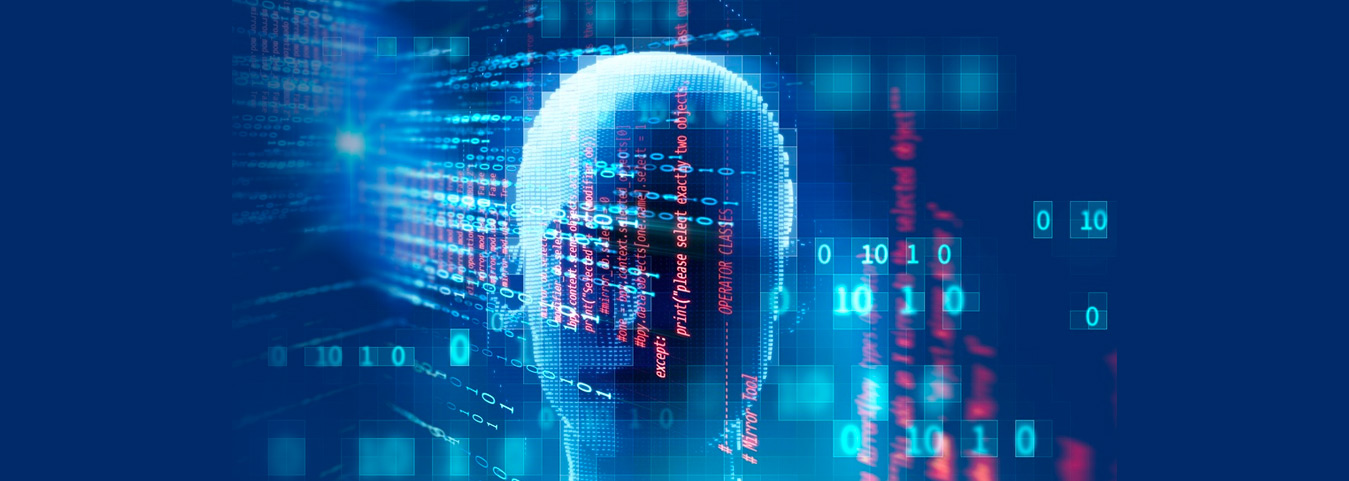
The Challenge
The company provides telecom services in major parts of North America and the UK, hence the business is very big, so is the data generated by their operation. The company wanted to have an AI-based monitoring and decision-making facility to identify key risks involved in their service such as to analyze call data, categorize it, identify the critical failure area and remediate the issue. By ensuring that the service does not suffer an already known issue and it will be easy to provide a seamless service.
Our Approach
The main idea behind the problem is to identify Good service from a Bad service. The critical part here is that because of so many factors to be considered before deciding on a service as Good or Bad, it is impossible to implement a rule-based decision-making system in place and there is risk of failure of such a system if the factors are going to change in the future, based on the nature of the service.
The second idea is to forecast the number of calls generated per minute based on various factors such as geography, season, trend, etc. By forecasting this the company will be able to allocate resources dynamically in an efficient manner and can automate subsequent down-stream processes. Our idea is
- To implement the unsupervised learning technique to find anomalies in service and identify a bad service.
- To use the RNN based Neural Network model to help forecasting call data
The goal is to provide the entire solution as a Data product where it will be easy to add such solutions in the future to this product.
The Solution
Based on our approach we implemented PCA, Isolation Forest and Autoencoder based anomaly detection models to identify anomalies from the good service. Hence there is a chance of high false-positive cases if we depend on a single model, we implemented two models in production and took cumulative inference for decision making. The time-series call data forecasting was achieved by training an LSTM model on historical volume data and to forecast for the desired time in the future. Notably, these two solutions are real-time which required a high level of optimization to accommodate the high frequency of incoming data. We deployed the models using Kubernetes and OKD deployment frameworks coupled with NVidia GPUs for high-performance model training. Additionally, AngularJS UI was developed to serve as a front-end for this application which will help the user monitor, schedule and take action right from the UI without worrying about the backend processes. Both the services are exposed as APIs in order to integrate any down-stream process for automation purposes.
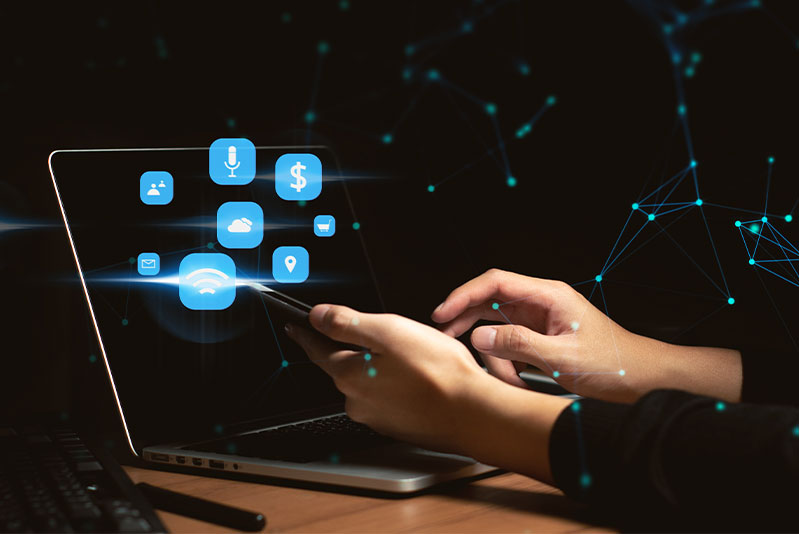